TELECOM LAB PROJECT
Radio Resource management in O-RAN
This project focused on the advancements of future B5G/6G networks. Those networks will introduce significant innovations, such as:
Higher frequency bands (mmWave, THz)
Cell-less deployment scheme.
Device-free deployment scheme.
Reconfigurable intelligent antennas.
These new radio environments present substantial challenges. It requires entirely new approaches to traditional tasks such as mobility optimization and resource allocation.
In this project, we address these challenges by developing advanced optimization and failure management algorithms that leverage predictions and context information (e.g., user location) to improve network performance.
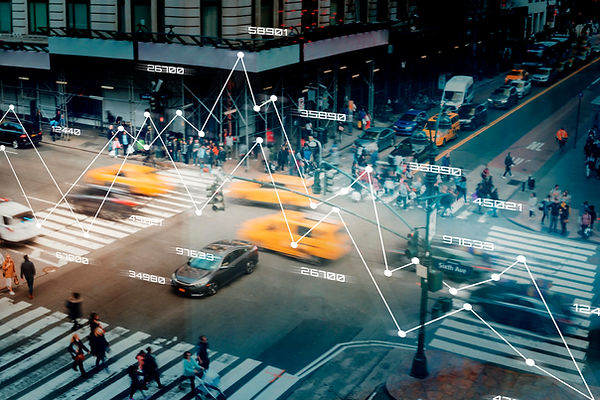
USE CASE
Mobility management is critical in telecommunications networks. It ensures seamless connectivity and optimal user experience. It involves intelligent coordination of handovers, session continuity, and resource allocation to support users in highly dynamic environments, such as urban areas with dense mobile traffic or high-speed transportation networks.
Current L3 handover mechanisms are primarily reactive, relying on historical measurement results or triggered events to initiate and execute handovers. While effective for low-mobility UEs in macro cell environments, this approach can struggle in scenarios with high UE mobility, dense micro cell deployments, or advanced services like XR.
Reactive schemes often lead to issues such as handover failures, radio link failures, Ping-Pong effects, throughput degradation, and improper timing of handovers. To enhance robustness, 3GPP has introduced Conditional Handover (Rel-16) and LTE HO (Rel-18) to reduce interruption times during frequent small-cell handovers. However, these methods remain inherently reactive by design.
INNOVATION DIRECTION
Our mobility management use case explores cutting-edge innovations by leveraging the regression and link prediction capabilities of Graph Neural Networks (GNNs). In the context of cellular networks, GNNs provide a powerful framework to model complex relationships between network entities, such as user equipment (UE), base stations, and radio links.
By applying GNNs, we aim to accurately predict critical radio link metrics, such as signal strength, interference levels, and handover success probabilities, as well as determine the most likely next cell for a given UE.
This proactive approach enables more intelligent and efficient handover decisions, reducing latency, minimizing handover failures, and enhancing overall performance.
By integrating these advanced AI techniques, we seek to redefine mobility management, ensuring seamless connectivity and superior user experiences in dynamic and high-density network environments.